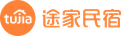
Tujia.com
Founded Year
2011Stage
Series E | AliveTotal Raised
$763.7MValuation
$0000Last Raised
$300M | 7 yrs agoAbout Tujia.com
Tujia.com offers online vacation rental services. It manages the properties listed on the website and works with real estate developers to rent out unsold inventory, including apartments, homestays, and villas. The company was founded in 2011 and is based in Beijing, China.
Loading...
Loading...
Expert Collections containing Tujia.com
Expert Collections are analyst-curated lists that highlight the companies you need to know in the most important technology spaces.
Tujia.com is included in 2 Expert Collections, including Unicorns- Billion Dollar Startups.
Unicorns- Billion Dollar Startups
1,258 items
Travel Technology (Travel Tech)
2,715 items
The travel tech collection includes companies offering tech-enabled services and products for tourists and travel players (hotels, airlines, airports, cruises, etc.). It excludes financial services and micro-mobility solutions.
Latest Tujia.com News
Nov 25, 2024
Ling Hou,1,2,* Ke Su,1,* Jinbo Zhao,1 Ting He,2 Yuanhong Li1 1Cardiovascular Disease Center, Central Hospital of Tujia and Miao Autonomous Prefecture, Enshi, Hubei Province, People’s Republic of China; 2Department of Central Hospital of Tujia and Miao Autonomous Prefecture, Hubei University of Medicine, Shiyan, Hubei, People’s Republic of China *These authors contributed equally to this work Background: Coronary heart disease (CHD) is a leading cause of mortality worldwide, with atrial fibrillation (AF) being a common complication. Chronic inflammatory responses play a significant role in the relationship between coronary artery disease and AF. This study aims to investigate the value of the multi-inflammatory index (MII) in predicting the occurrence of atrial fibrillation in patients with coronary heart disease. Methods: A retrospective analysis was conducted on patients who visited our hospital from January 1, 2020, to December 31, 2023, including a total of 1392 patients. Clinical data and laboratory results were collected. Feature selection was performed using the Boruta algorithm. Five machine learning models were constructed: Logistic Regression, Decision Tree, Elastic Net, Light Gradient Boosting Machine (LightGBM), and Multilayer Perceptron. Model performance was evaluated using five-fold cross-validation. SHAP values were utilized to analyze feature importance and model interpretability. Results: The study included 1302 patients without AF and 90 patients with AF. Patients with AF had significantly higher MII compared to those without AF (10.02 vs 4.79). Thirteen variables most related to AF occurrence were selected using the Boruta algorithm. The LightGBM model outperformed others, showing the highest accuracy and calibration in both training and test sets. In the training set, LightGBM achieved an AUC of 0.958, accuracy of 0.851, and sensitivity of 0.943, while in the testing set, it achieved an AUC of 0.757 and accuracy of 0.821. SHAP analysis indicated that age, heart rate, and MII were the primary predictors of AF occurrence. Conclusion: The LightGBM model demonstrated adequate sensitivity and accuracy. The multi-inflammatory index plays a crucial role in predicting atrial fibrillation in patients with coronary heart disease. Keywords: atrial fibrillation, coronary heart disease, multi-inflammatory index, machine learning models, light gradient boosting machine Background Coronary artery disease (CAD) remains a leading cause of mortality in both developed and developing countries. 1 Atrial fibrillation (AF) is a persistent cardiac arrhythmia characterized by rapid and irregular electrical activity replacing the normal, organized atrial contraction. 2 AF can lead to adverse outcomes such as stroke, exacerbated heart failure, thromboembolism, cognitive impairment, and decreased quality of life. 3 According to recent global statistics, AF affects approximately 33 million people worldwide, and its prevalence is expected to increase as populations age, particularly among patients with underlying cardiovascular conditions like CAD. Numerous studies have shown that CAD patients are more susceptible to developing AF. 4 Chronic inflammation, often accompanying coronary artery lesions, may exacerbate cardiac electrical instability, thereby increasing the risk of AF. 5 Moreover, the presence of AF can worsen CAD symptoms and elevate the risk of acute coronary events, creating a vicious cycle. 6 The multi-inflammatory index (MII), a composite inflammation score based on various markers such as C-reactive protein (CRP), neutrophils, and lymphocytes, offers a comprehensive reflection of the body’s inflammatory status. 7 MII has shown significant potential in cardiovascular disease (CVD) risk assessment. 8 As a simple and accessible metric, MII holds promise for broad clinical application, aiding in risk evaluation and personalized treatment of CVD. Despite advances in treatment and prevention, the predictive accuracy of existing models for AF in CAD patients remains suboptimal, further highlighting the need for innovative approaches to risk prediction. AF is a common complication in CAD patients, and early prediction and intervention are crucial for improving prognosis. 9 This study aims to investigate the predictive value of MII for AF in CAD patients. We will employ machine learning (ML) methods to build and train predictive models, analyzing MII and other clinical indicators to assess their predictive capability for AF risk. This ML model will help clinicians accurately identify high-risk patients, optimize treatment strategies, and improve patient outcomes. Methods We retrospectively selected patients who visited our hospital between January 1, 2020, and December 31, 2023. Patients diagnosed with CAD, defined as coronary artery stenosis ≥ 50% by angiography, 10 were included. Patients with a history of AF (paroxysmal, persistent or permanent), other cardiac diseases, malignancies, or missing CRP data were excluded ( Figure 1 ). The study adheres to medical research guidelines, has been approved by the Ethics Committee of Enshi Tujia and Miao Autonomous Prefecture Central Hospital, and complies with the Helsinki Declaration. Given the retrospective nature of the study, the ethics committee did not require informed consent from all patients, but mandated confidentiality of medical records. The AF diagnosis followed the 2023 ACC/AHA/ACCP/HRS Atrial Fibrillation Diagnosis and Management Guidelines. 11 A total of 1392 patients were selected and split into training and validation sets in a 7:3 ratio. Figure 1 Establishing the modeling process. Data Collection The collected clinical data comprised various parameters, including demographic information such as gender, age, and comorbidities (hypertension, diabetes, stroke, hyperlipidemia), as well as details on smoking and drinking history. Additionally, laboratory indicators encompassed measurements of white blood cells, neutrophils, lymphocytes, monocytes, hemoglobin, platelet distribution width, platelets, mean platelet volume, red cell distribution width, direct bilirubin, total cholesterol (TC), triglycerides (TG), platelet count (PLT), high-density lipoprotein cholesterol (HDL-c), low-density lipoprotein cholesterol (LDL-c), creatinine, glucose, uric acid, and potassium ions. Examination indicators included heart rate, systolic and diastolic blood pressure, and ejection fraction (EF%). The assessment of coronary lesions examined the left main, left anterior descending, circumflex, and right coronary artery, as well as the presence of multi-vessel disease. The MII index was derived by multiplying the neutrophil-to-lymphocyte ratio with CRP levels. 12 Feature Selection Boruta creates shadow features for each variable and uses a random forest to compute the importance scores of original and shadow features. Compared to traditional variable screening methods, Boruta offers more comprehensive, accurate, and efficient variable selection. ML Model Development and Evaluation We developed five common ML models: logistic regression, decision tree, elastic net, light gradient boosting machine (LightGBM), and multilayer perceptron. Five-fold cross-validation (CV) was used to evaluate model generalization. Optimal hyperparameters were determined via grid search. Performance metrics included F1 score, accuracy, recall, precision, and area under the curve (AUC). These metrics assessed the overall performance and clinical applicability of the models. Feature Importance and Model Interpretability We selected the best-performing ML model for feature importance analysis to determine the impact of features on prediction. Feature importance was calculated based on the model’s internal mechanisms. We analyzed the top five features and visualized their importance. Additionally, SHAP (SHapley Additive exPlanations) was used for interpreting ML model results, revealing the contribution and impact of each feature on AF risk prediction. Statistical Analysis Normally distributed data were expressed as mean ± standard deviation and compared using t-tests; non-normally distributed data were expressed as medians and compared using non-parametric tests. Categorical data were presented as counts and percentages, and comparisons used χ2 tests. A significance level of α = 0.05 was set, with P < 0.05 indicating statistical significance. Statistical analysis was conducted using Python 3.9 and R 4.3.2. Results Baseline Characteristics Table 1 presents the baseline characteristics of study participants. The study included 1302 non-AF CAD patients and 90 AF patients. Significant differences were found in age, heart rate, hemoglobin, platelet count, mean platelet volume, MII, aspartate aminotransferase, direct bilirubin, total cholesterol, triglycerides, LDL, and creatinine levels between the two groups. AF patients had a significantly lower ejection fraction (63 vs 59). AF patients had higher incidences of hyperlipidemia and stroke; left main coronary artery involvement was more likely to lead to AF. No significant differences were found in gender, hypertension, diabetes, smoking, drinking, multi-vessel disease, white blood cell count, HDL, uric acid, potassium, and glucose levels between the two groups. Table 1 Descriptive Characteristics of Overall Participants Feature Selection Using Boruta Boruta confirmed the importance of 13 variables most associated with AF risk in CAD patients ( Figure 2 ). Despite its lower Z-score, gender was included based on previous studies and clinical experience. Figure 2 The process of feature selection based on Boruta’s algorithm. Model Comparison Table 2 shows the performance comparison of logistic regression, decision tree, elastic net, LightGBM, and multilayer perceptron models on training and validation sets. Figure 3 visually presents the performance comparison across different metrics. The LightGBM model demonstrated the best performance, with the highest accuracy and calibration. Figure 4 shows calibration curves and Brier scores (BS) for different ML models in the training set. The LightGBM model’s calibration curve was closest to the ideal, with the lowest BS of 0.044, indicating high predictive accuracy. Overall, LightGBM exhibited the best performance and stability among all ML models. Table 2 Model Performance Comparison Figure 3 ROC curve of the model. Figure 4 Comparison of calibration curves of each model. Explaining LightGBM Models Using SHAP The SHAP method is employed to assess the contribution of each predictor variable to the predictions made by the LightGBM model. Figure 5 displays the variable importance plot, where variables are sorted in descending order of importance. Among all predictors, the mean value of age exhibits the highest predictive value, followed closely by heart rate and MII. To further examine the positive or negative correlations between predictors and the target outcome, we utilize SHAP values to identify risk factors associated with mortality. As illustrated in Figure 6 , the horizontal position shows whether the value is associated with a higher or lower prediction, while color indicates whether the variable is high (red) or low (blue) for that observation. We observe that increases in age, heart rate, and MII contribute positively to the prediction of atrial fibrillation incidence. Figure 5 The weights of variables importance. Figure 6 The SHapley Additive exPlanation (SHAP) values. SHAP Force Plot for Individual Predictions Figure 7 presents a SHAP force plot for an individual sample, highlighting the impact of each feature on the model’s output. SHAP values reflect the relevant features for predicting atrial fibrillation in a specific coronary heart disease patient, along with the contribution of each feature to the prediction. The baseline value, E[f(x)], represents the average prediction across all samples, whereas the final prediction, f(x), is the output for the particular sample. Positive contributions (yellow) indicate that the feature increases the model’s prediction, while negative contributions (red) indicate that the feature decreases the prediction. For instance, being 82 years old, having an MII value of approximately 1.84, and a glucose level above 5.33 are factors that increase the risk of atrial fibrillation. Conversely, a heart rate of 78 beats per minute is associated with a reduced risk of atrial fibrillation. Figure 7 SHAP Individual Force Plots. Discussion In our study, we found that MII levels were significantly higher in coronary heart disease (CHD) patients who developed AF compared to those who did not (10.02 vs 4.79). AF patients exhibited poorer cardiac function, with a notably lower ejection fraction than those without AF. Using the Boruta algorithm for feature selection, we identified the significance of 13 variables most relevant to the risk of AF in CHD patients. Among the models compared, the LightGBM demonstrated superior performance on both training and test sets, showcasing the highest accuracy and calibration, indicating its strong predictive capability for AF occurrence in CHD patients. Furthermore, we employed SHAP to interpret the predictions made by the LightGBM model. Advanced age, increased heart rate, and higher MII levels were positively associated with the onset of AF. The SHAP summary plot illustrated the impact of each feature on the model’s output, elucidating the direction of influence of different feature values on AF risk. MII is a novel prognostic indicator developed by Gardini et al in 2020, initially showing a close correlation with the prognosis of colorectal cancer patients. 13 Subsequent studies have reinforced the prognostic and predictive value of MII in various clinical settings. 14–18 Neutrophils play a crucial role in the onset and progression of AF by releasing pro-inflammatory factors and reactive oxygen species (ROS). They can trigger and sustain local inflammatory responses, leading to structural changes in the atria and promoting AF development. 19 Lymphocytes, particularly T cells, also play a significant role in cardiac inflammatory responses, influencing atrial electrophysiological stability through the regulation of immune responses and release of inflammatory mediators. 20 CRP, an acute-phase protein, typically indicates the presence of inflammation or infection when elevated. CRP can alter the electrophysiological properties of myocardial cells by binding to phosphatidylcholine on the cell membrane, affecting sodium (Na+) and calcium (Ca2+) exchange, and leading to atrial electrophysiological remodeling that promotes AF. 21–23 By calculating the MII value, we can comprehensively consider changes in neutrophils, lymphocytes, and CRP, thereby providing a more thorough assessment of the inflammatory state and immune health in AF patients. This is crucial for predicting AF occurrence in CHD patients and for developing individualized treatment plans. Through the analysis of the importance of predictive variables and their association with AF occurrence, we identified key predictors of AF, with increased MII levels promoting AF in CHD patients. Yun et al’s study confirmed that age is a risk factor for AF, 24 controlling heart rate can prevent AF, 25 and higher DBIL levels lead to AF recurrence. 26 These findings are consistent with our study and, in conjunction with our results, underscore the predictive capability of MII for AF occurrence in CHD patients. Furthermore, a more detailed comparison with similar research could better contextualize the contribution of our study to the broader understanding of AF risks in CHD patients. While existing studies have emphasized individual inflammatory markers or demographic factors, our study highlights the predictive power of MII as a comprehensive inflammatory index, offering a novel perspective on the interplay between inflammation and AF development. Despite the strengths of our study, there are limitations that should be acknowledged. Our study has limitations, including its retrospective nature, which may provide a lower evidence level, and a single-center sample, limiting generalizability. The retrospective design may introduce bias, and future prospective studies with larger, multicenter populations are warranted to validate our findings. Additionally, the complex mechanisms between inflammation and AF warrant further exploration. External validation of the LightGBM model is needed, which will further confirm the model’s robustness and generalizability across different populations and settings. Conclusion The LightGBM model demonstrated sufficient sensitivity and accuracy, with consistent associations between MII levels and AF in CAD patients. However, external validation and further investigation into AF mechanisms are required. Disclosure
Tujia.com Frequently Asked Questions (FAQ)
When was Tujia.com founded?
Tujia.com was founded in 2011.
Where is Tujia.com's headquarters?
Tujia.com's headquarters is located at Floor 5 Building 148 , Beijing.
What is Tujia.com's latest funding round?
Tujia.com's latest funding round is Series E.
How much did Tujia.com raise?
Tujia.com raised a total of $763.7M.
Who are the investors of Tujia.com?
Investors of Tujia.com include Ctrip, All-Stars Investment, China Renaissance, Glade Brook Capital, G Street Capital and 10 more.
Who are Tujia.com's competitors?
Competitors of Tujia.com include AsiaYo, Gathern, XiaoZhu, Airbnb, Ziroom and 7 more.
Loading...
Compare Tujia.com to Competitors

XiaoZhu is a Beijing-based short-term lodging sharing platform. It provides lodging sharing services in over 130 cities in China. It was founded in 2012 and is based in Beijing, China.
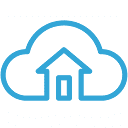
TRAIWAN is a hospitality service provider focused on offering lodging options across various popular travel destinations. The company's main services include a platform for booking distinctive homestays, private villas, family-friendly accommodations, camping areas, camper vans, backpacker hostels, and more, with a user-friendly interface and multiple payment options. It primarily serves the tourism and travel industry by facilitating easy and diverse lodging bookings. It is based in Taiwan.

Mofang Living operates in the public rental housing sector in China, focusing on providing living spaces. The company offers furnished housing solutions that are flexible and affordable for consumers. Mofang Living caters to a diverse customer base, including business travelers, expatriates, and urban creative professionals, by providing short-term rental products and a community living environment. It was founded in 2009 and is based in Shanghai, Shanghai.

Locals offers a mobile homestay rental platform that enables travelers to book boutique bed and breakfasts. The company offers a management platform that helps landlords in the design, renovation, promotion, reception, and operation of their lodgings. It was founded in 2015 and is based in Beijing, China.
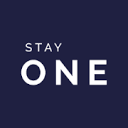
Stay One Degree operates in the holiday home rental sector, providing properties. The company offers holiday homes that are vetted and adhere to quality standards. Stay One Degree has partnerships with hotel groups. It was founded in 2017 and is based in Hong Kong.
StayList is a search engine specializing in homestay accommodations within the travel and hospitality industry. The platform aggregates listings from multiple sites, allowing users to efficiently find and compare homestay options worldwide. It provides various filters to refine search results by accommodation type, amenities, and location. It was founded in 2018 and is based in Tokyo, Japan.
Loading...