
InstaDeep
Founded Year
2014Stage
Incubator/Accelerator - VII | AliveTotal Raised
$113.65MAbout InstaDeep
InstaDeep focuses on delivering artificial intelligence (AI)-powered decision-making systems for various enterprise sectors. The company offers solutions that leverage computing, deep learning, and reinforcement learning to address challenges in industries such as biology, logistics, electronic design, and energy. Its products and services are designed to help the capabilities of businesses by providing advanced AI tools and research insights. InstaDeep was formerly known as Digital Ink Group. It was founded in 2014 and is based in London, United Kingdom.
Loading...
InstaDeep's Product Videos
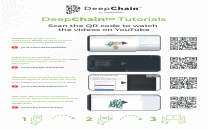
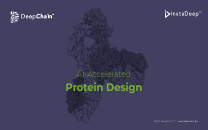
ESPs containing InstaDeep
The ESP matrix leverages data and analyst insight to identify and rank leading companies in a given technology landscape.
The AI molecular design platforms market includes software platforms that utilize AI/ML to screen vast chemical spaces and predict molecular properties for drug development. These solutions analyze structure-activity relationships, optimize drug-like properties (ADMET), and enable multi-parameter optimization of lead compounds. Core functionalities include virtual screening, de novo drug design, a…
InstaDeep named as Leader among 15 other companies, including XtalPi, Isomorphic Laboratories, and Iktos.
InstaDeep's Products & Differentiators
DeepChain
AI-Accelerated Drug Design
Loading...
Research containing InstaDeep
Get data-driven expert analysis from the CB Insights Intelligence Unit.
CB Insights Intelligence Analysts have mentioned InstaDeep in 6 CB Insights research briefs, most recently on Dec 11, 2024.
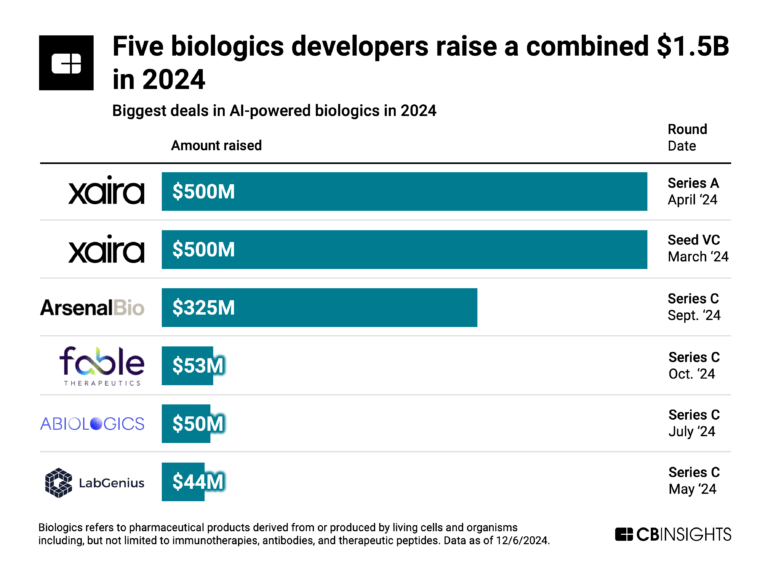
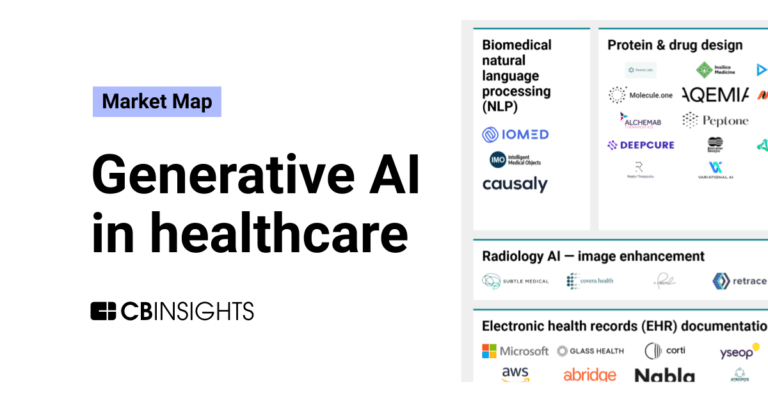
Jan 11, 2024
The generative AI in healthcare market map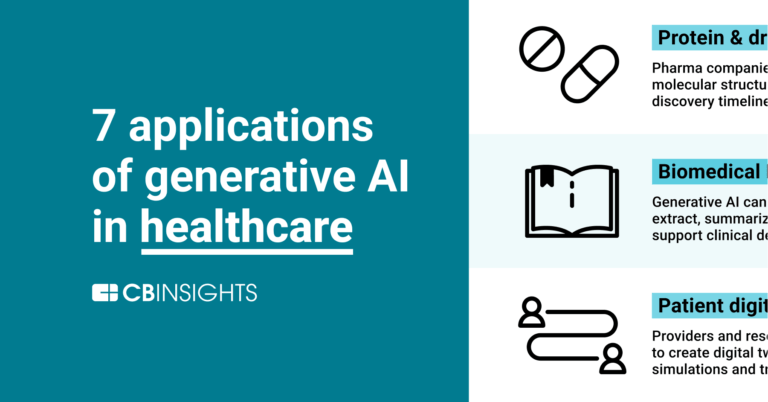
May 9, 2023
7 applications of generative AI in healthcare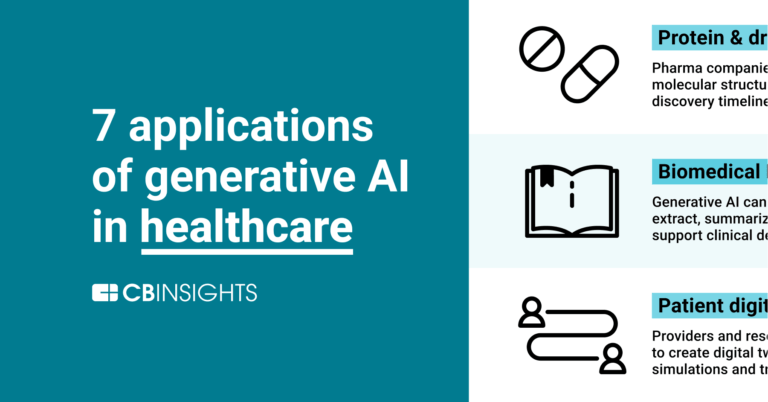
May 9, 2023
7 applications of generative AI in healthcareExpert Collections containing InstaDeep
Expert Collections are analyst-curated lists that highlight the companies you need to know in the most important technology spaces.
InstaDeep is included in 5 Expert Collections, including Artificial Intelligence.
Artificial Intelligence
9,504 items
Companies developing artificial intelligence solutions, including cross-industry applications, industry-specific products, and AI infrastructure solutions.
AI 100
300 items
The winners of the 4th annual CB Insights AI 100.
Semiconductors, Chips, and Advanced Electronics
7,309 items
Companies in the semiconductors & HPC space, including integrated device manufacturers (IDMs), fabless firms, semiconductor production equipment manufacturers, electronic design automation (EDA), advanced semiconductor material companies, and more
Generative AI
1,298 items
Companies working on generative AI applications and infrastructure.
AI in drug discovery
504 items
Companies using AI to advance therapeutic discovery, categorized into: platforms (primary product is software) and discovery engines (primary product is therapeutics). Additional funnel descriptions reflect how companies are applying AI.
InstaDeep Patents
InstaDeep has filed 8 patents.
The 3 most popular patent topics include:
- artificial neural networks
- electronic design
- machine learning
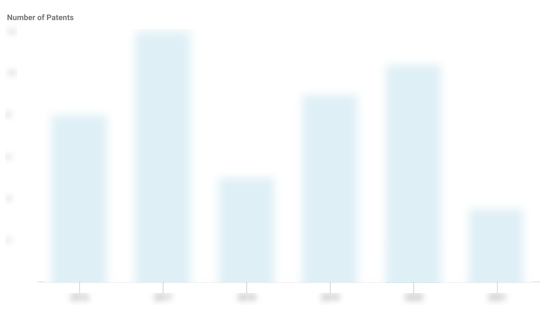
Application Date | Grant Date | Title | Related Topics | Status |
---|---|---|---|---|
11/30/2021 | 12/3/2024 | Electronic design, Artificial neural networks, Electronic design automation, Electronic engineering, Integrated circuits | Grant |
Application Date | 11/30/2021 |
---|---|
Grant Date | 12/3/2024 |
Title | |
Related Topics | Electronic design, Artificial neural networks, Electronic design automation, Electronic engineering, Integrated circuits |
Status | Grant |
Latest InstaDeep News
Feb 21, 2025
resilience Menu February 19, 2025 The field of plant biotechnology is undergoing a profound transformation. The rise of generative artificial intelligence (AI) tools is fundamentally reshaping the way genetic engineering is conducted. AI-driven genetic engineering may be vulnerable to well-known limitations of AI, such as the black box effect, hallucinations and data errors, raising concerns that plants with undesirable traits could be engineered and released into the environment. How should the EU respond? Benny Haerlin and Franziska Achterberg of Save our Seeds summarise findings of the organisation’s report When Chatbots Breed New Plant Varieties. An Artificial Intelligence (AI) program predicting the three-dimensional structure of more than 200 million proteins and another one using this information to design “new to nature”, hitherto unseen functional proteins, have shared the 2024 Nobel Prize for Chemistry. The new US president last week announced a 500 billion Dollar investment in the biggest AI infrastructure ever – while repealing all safety orders of his predecessor regarding AI. A few days later the Chinese AI startup DeepSeek demonstrated that even more computing power can be generated at a fraction of the US Tech companies’ prices. To this backdrop, the convergence of genetic engineering techniques with machine learning algorithms, processing exponentially growing amounts of data at ever increasing speed, is about to disrupt and transform agriculture, food and any other bio-production. The potential of this “new wave” of technologies is breathtaking – and so are the risks associated with it. The European GMO watchdog “Save Our Seeds” new report – When Chatbots Breed New Plant Varieties – describes the volatile state of the art of using AI for generating new plants. The context is the proposed deregulation of genetically modified plants by the Commission and the European Parliament. Is it smart to intentionally give up control over the release of modern GM plants at the very moment when AI technology will transform this technology to the extent that human intelligence may no longer be in the driving seat? AI models trained in the ‘languages’ of biology Developers have used the AI architectures of the large language models used in chatbots like ChatGPT or image generators like DALL-E and trained them in the ‘languages’ of biology – specifically, protein and genome sequences. This has become possible because an immense wealth of data on DNA and RNA sequences, proteins and metabolites of plants has become available in recent years. These data now form the raw material that allows the development of generative AI for genetic engineering. The resulting AI tools are both descriptive and generative. Deep learning algorithms can analyse biological data and make predictions. Additionally, they already allow for the design of functional DNA, RNA, and protein sequences, including ‘new-to-nature’ sequences never observed in nature. Depending on the type of ‘language’ used to train the models, several distinct categories have emerged: Protein Models: These models can analyse proteins, simulate their interactions and redesign their functions. One of the most notable tools is Google’s Alphafold. In recognition of its groundbreaking contribution to the field, Demis Hassabis, head of Google’s AI division, along with two colleagues, was awarded the 2024 Nobel Prize in Chemistry for the development of this model. DNA Models: The first large language models trained on DNA sequences emerged in 2021. Today, four models exist that have been trained specifically on plant DNA. The most advanced of these is AgroNT, a collaboration between Google and Instadeep, was released in late 2023. This model was trained on 10 million genome sequences from 48 plant species. RNA Models: AI models trained on human RNA sequences already exist, while plant-based RNA models will be developed in the near future. Models like scGPT, based on single-cell RNA sequencing (scRNA-seq) data, are considered particularly promising for advancing plant science and breeding. Multimodal Models: Rather than working with a single type of data, AI developers are now working on integrating multimodal models that can process various kinds of biological data. In 2024, Instadeep and BioNTech presented the first multimodal AI architecture capable of connecting DNA, RNA, and protein data. Use of AI in the genetic engineering of plants Gene editing today primarily relies on the CRISPR-Cas method. Specific AI tools are now available to enhance the process. These tools assist researchers in identifying optimal targets, suggesting the most effective sequences for the guide RNA and selecting the most suitable CRISPR cutting enzymes. The use of these tools is already commonplace in GM labs around the world and can make gene editing with CRISPR more precise and efficient. Arguably, AI tools have also expanded the capabilities of CRISPR beyond traditional applications. Developers can now induce not only loss-of-function mutations (so called knockouts which account for most CRISPR-Cas edited plants so far) but also mutations that make it possible to control levels of gene expression. This advancement, known as quantitative trait engineering, is made possible largely thanks to AI. The ability to control gene expression opens the potential to influence complex quantitative traits, offering new possibilities for plant genetic engineering. Here are some examples of how AI models can enhance the genetic engineering of plants: The US company TreeCo is working to engineer poplar trees with reduced lignin production, which could simplify paper production. The company has developed an AI tool that predicts how changes to 21 genes involved in lignin synthesis will impact the trees’ wood composition, growth rate, and other traits. The tool identifies over 69,000 potential editing strategies for these genes, narrowing them down to the best options through computer simulations. Based on this analysis, TreeCo is experimentally testing the seven most promising gene-editing combinations in the poplar genome. Another US company, Inari, is developing maize varieties with reduced height and increased leaf biomass. The company uses an AI tool to predict how mutations in promoter regions will influence plant characteristics. Currently, Inari is conducting field trials of a short-growing maize variety in Belgium. Academic researchers have leveraged the Alphafold protein model to redesign patatin, a protein naturally found in potatoes. The AI-generated version of patatin is designed to improve the viscosity and nutritional properties of dough made from potato flour. The researchers aim to incorporate this AI-enhanced protein into the potato genome to achieve these improvements. Large seed companies such as Corteva, Bayer, BASF, and Syngenta are increasingly using AI tools in their genetic engineering programmes. To complement their in-house AI expertise, these companies are also partnering with a diversity of specialised firms. What’s next? The development of generative AI models for gene editing is still in its infancy. Many of the design tools currently available are so new that there is insufficient experimental data to fully assess the performance of their algorithms. However, it is already clear that these tools are creating new design possibilities that go beyond natural limits. In the coming years, the quality of data acquisition techniques, the volume of data collected and the computing power to process them are expected to grow exponentially. The descriptive and generative capabilities of AI will constantly grow. Experience with large language models trained with microbial DNA sequences demonstrates the potential that genomic AI tools could have. One such model, EVO, can artificially generate sequences on the scale of entire microbial genomes, according to its developers. What could go wrong? The convergence of AI and genetic engineering raises a number of concerns, including, but not limited to: Lower skill threshold. Traditionally, plant genetic engineering has been the domain of highly trained professionals. However, with the advent of AI tools, gene editing could soon become accessible to students, computer scientists, entrepreneurs, or even DIY biologists. Black box. Generative AI models produce predictions or recommendations without providing insight into how or why they arrived at those conclusions. In sensitive areas such as plant genetic engineering, where the products reproduce and interact in nature, and the consequences can affect public health and the environment, the lack of comprehension and reproducibility is particularly concerning. Hallucinations. Generative AI models sometimes produce outputs that seem plausible but are factually incorrect or irrelevant. ChatGPT users are familiar with such glitches, reminding them of the fact that AI models have no concept for factuality, truth or even responsibility. The frequency and context in which these ‘hallucinations’ occur, and how to mitigate them, remain unclear. The bigger the data processed, the lower the probability of immediate detection and the more sensitive the use of the results the higher the concerns. Data distortion. The outputs and predictions of generative AI models are shaped by the data used to train them. If these data contain errors or biases — whether originating from the biological systems themselves or from human curators — they will be reflected in the model’s results. EU set to deregulate AI-designed plants At this critical moment, the EU is moving to relax regulatory requirements for the commercialisation of genetically engineered plants. In a proposal from July 2023, the European Commission suggests that plants modified with gene-editing tools like CRISPR-Cas should be treated similarly to conventionally bred plants. Specifically, plants with no more than 20 targeted changes to their genome would be exempt from EU GMO regulations and could be marketed without prior risk assessment, detection methods, traceability, or consumer labelling. Numerous scientists, authorities, and NGOs have criticized the Commission’s proposal. The Federal Agency for Nature Conservation (BfN) in Germany highlighted that most gene-edited plants would be released into the environment without any risk assessment, warning that even small genetic changes could have significant consequences and pose high risks. The French food authority ANSES argued that the threshold of 20 nucleotides is not suitable for demonstrating equivalence to conventionally bred plants. In contrast, the European Food Safety Authority (EFSA) defended the Commission’s proposal. The use of generative AI models may allow developers to fully exploit the ‘design space’ of 20 genetic modifications to, for instance, engineer plants producing insect toxins. Sticking to the European Commission’s “magic number”, however, no tests would be required to assess the plant’s impact on wild species and eco-systems. The way forward Instead of relaxing regulatory standards, Save Our Seeds demands that present minimum requirements for the release of GMOs should be upheld. Further, a thorough assessment and discussion of the potential new risks and hazards associated with AI systems producing genetically engineered plants as well as other organisms should be initiated. Making GM as well as AI regulations fit for future should ensure that the AI models used are reliable and capable of making safe recommendations, while preserving human comprehension, oversight, and decision-making at critical stages of the genetic engineering process. The full report written by Benno Vogel, an SOS press release and a summary are available in English and in German at the organisation’s website.
InstaDeep Frequently Asked Questions (FAQ)
When was InstaDeep founded?
InstaDeep was founded in 2014.
Where is InstaDeep's headquarters?
InstaDeep's headquarters is located at 5 Merchant Square, London.
What is InstaDeep's latest funding round?
InstaDeep's latest funding round is Incubator/Accelerator - VII.
How much did InstaDeep raise?
InstaDeep raised a total of $113.65M.
Who are the investors of InstaDeep?
Investors of InstaDeep include African Unicorns, BioNTech, NVIDIA Inception Program, Chimera Investments, G42 and 13 more.
Who are InstaDeep's competitors?
Competitors of InstaDeep include Variational AI, Iktos, Isomorphic Laboratories, Insilico Medicine, Aqemia and 7 more.
What products does InstaDeep offer?
InstaDeep's products include DeepChain.
Who are InstaDeep's customers?
Customers of InstaDeep include BioNTech.
Loading...
Compare InstaDeep to Competitors

Cradle operates in protein engineering within the biotechnology sector. The company has a platform that employs machine learning to design variants of protein sequences, aiding the protein engineering process for various applications such as therapeutics, chemicals, materials, and food. Cradle's services are aimed at biotech teams involved in research and development projects. It was founded in 2021 and is based in Delft, Netherlands.
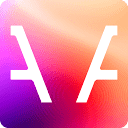
Aqemia focuses on transforming the drug discovery process. The company offers technology that combines quantum-inspired physics and machine learning to scale the drug discovery process, generating a rapidly growing pipeline of new drug candidates. It primarily sells to the pharmaceutical industry. It was founded in 2019 and is based in Paris, France.
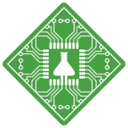
Insilico Medicine is a biotechnology company that focuses on artificial intelligence in drug discovery and development. The company provides AI platforms for multi-omics target discovery, deep biology analysis, automated drug design, and predicting outcomes in clinical trials. The technologies are intended to assist in the process of discovering and developing new medications for various diseases. It was founded in 2014 and is based in Pudong, China.
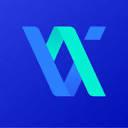
Variational AI is a company focused on drug discovery utilizing generative AI within the biopharmaceutical sector. The company offers Enki, a platform that generates novel drug-like small molecules based on user-defined therapeutic product profiles (TPP) without requiring prior data. Variational AI primarily serves the biopharmaceutical industry, collaborating with partners to develop new therapeutics. It was founded in 2019 and is based in Vancouver, Canada.
YDS Pharmatech is a company focused on drug discovery in the pharmaceutical industry. The company offers a platform for the design of new drug candidates, utilizing structure-based de novo drug design and evolutionary AI to accelerate the drug discovery process. YDS Pharmatech primarily serves the pharmaceutical industry. It was founded in 2020 and is based in Albany, New York.

Biomatter is a synthetic biology company that focuses on creating new proteins for various applications within the health and sustainable manufacturing sectors. The company offers a proprietary intelligent architecture platform that develops proteins with the capabilities of traditional engineering methods. Biomatter was formerly known as Biomatter Designs. It was founded in 2018 and is based in Vilnius, Lithuania.
Loading...