
DevRev
Founded Year
2020Stage
Series A | AliveTotal Raised
$150.8MValuation
$0000Last Raised
$100.8M | 7 mos agoMosaic Score The Mosaic Score is an algorithm that measures the overall financial health and market potential of private companies.
+31 points in the past 30 days
About DevRev
DevRev specializes in AI-native platforms and applications for the SaaS industry. Its main offerings include modern CRM apps for support, product, and growth teams, designed to streamline collaboration, analytics, and customer engagement. Its products are tailored to enhance the customer experience, automate product management, and provide advanced analytics for decision-making. It was founded in 2020 and is based in Palo Alto, California.
Loading...
Loading...
Expert Collections containing DevRev
Expert Collections are analyst-curated lists that highlight the companies you need to know in the most important technology spaces.
DevRev is included in 3 Expert Collections, including Unicorns- Billion Dollar Startups.
Unicorns- Billion Dollar Startups
1,258 items
Artificial Intelligence
7,212 items
Generative AI
1,298 items
Companies working on generative AI applications and infrastructure.
DevRev Patents
DevRev has filed 4 patents.
The 3 most popular patent topics include:
- agile software development
- product lifecycle management
- product management
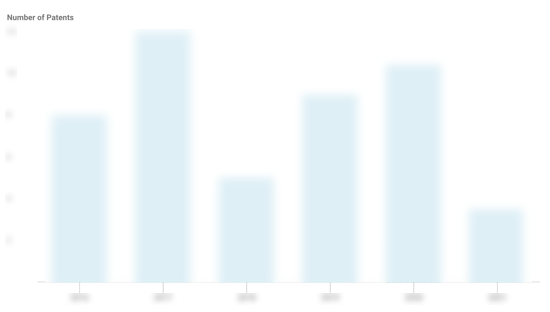
Application Date | Grant Date | Title | Related Topics | Status |
---|---|---|---|---|
2/11/2022 | 10/8/2024 | Grant |
Application Date | 2/11/2022 |
---|---|
Grant Date | 10/8/2024 |
Title | |
Related Topics | |
Status | Grant |
Latest DevRev News
Jan 25, 2025
OpenSearch Vector Engine is now disk-optimized for low cost, accurate vector search Like Views: 1 OpenSearch Vector Engine can now run vector search at a third of the cost on OpenSearch 2.17+ domains. You can now configure k-NN (vector) indexes to run on disk mode, optimizing it for memory-constrained environments, and enable low-cost, accurate vector search that responds in low hundreds of milliseconds. Disk mode provides an economical alternative to memory mode when you don’t need near single-digit latency . In this post, you’ll learn about the benefits of this new feature, the underlying mechanics, customer success stories, and getting started. Overview of vector search and the OpenSearch Vector Engine Vector search is a technique that improves search quality by enabling similarity matching on content that has been encoded by machine learning (ML) models into vectors (numerical encodings). It enables use cases like semantic search, allowing you to consider context and intent along with keywords to deliver more relevant searches. OpenSearch Vector Engine enables real-time vector searches beyond billions of vectors by creating indexes on vectorized content. You can then run searches for the top K documents in an index that are most similar to a given query vector, which could be a question, keyword, or content (such as an image, audio clip, or text) that has been encoded by the same ML model. Tuning the OpenSearch Vector Engine Search applications have varying requirements in terms of speed, quality, and cost. For instance, ecommerce catalogs require the lowest possible response times and high-quality search to deliver a positive shopping experience. However, optimizing for search quality and performance gains generally incurs cost in the form of additional memory and compute. The right balance of speed, quality, and cost depends on your use cases and customer expectations. OpenSearch Vector Engine provides comprehensive tuning options so you can make smart trade-offs to achieve optimal results tailored to your unique requirements. You can use the following tuning controls: Algorithms and parameters – This includes the following: Hierarchical Navigable Small World (HNSW) algorithm and parameters like ef_search, ef_construct, and m Inverted File Index (IVF) algorithm and parameters like nlist and nprobes Exact k-nearest neighbors (k-NN), also known as brute-force k-NN (BFKNN) algorithm Engines – Facebook AI Similarity Search (FAISS), Lucene, and Non-metric Space Library (NMSLIB). Compression techniques – Scalar (such as byte and half precision), binary, and product quantization Similarity (distance) metrics – Inner product, cosine, L1, L2, and hamming Vector embedding types – Dense and sparse with variable dimensionality Ranking and scoring methods – Vector, hybrid (combination of vector and Best Match 25 (BM25) scores), and multi-stage ranking (such as cross-encoders and personalizers) You can adjust a combination of tuning controls to achieve a varying balance of speed, quality, and cost that is optimized to your needs. The following diagram provides a rough performance profiling for sample configurations. Tuning for disk-optimization With OpenSearch 2.17+, you can configure your k-NN indexes to run on disk mode for high-quality, low-cost vector search by trading in-memory performance for higher latency. If your use case is satisfied with 90th percentile (P90) latency in the range of 100–200 milliseconds, disk mode is an excellent option for you to achieve cost savings while maintaining high search quality. The following diagram illustrates disk mode’s performance profile among alternative engine configurations. Disk mode was designed to run out of the box, reducing your memory requirements by 97% compared to memory mode while providing high search quality. However, you can tune compression and sampling rates to adjust for speed, quality, and cost. The following table presents performance benchmarks for disk mode’s default settings. OpenSearch Benchmark (OSB) was used to run the first three tests, and VectorDBBench (VDBB) was used for the last two. Performance tuning best practices were applied to achieve optimal results. The low scale tests (Tasb-1M and Marco-1M) were run on a single r7gd.large data node with one replica. The other tests were run on two r7gd.2xlarge data nodes with one replica. The percent cost reduction metric is calculated by comparing an equivalent, right-sized in-memory deployment with the default settings. These tests are designed to demonstrate that disk mode can deliver high search quality with 32 times compression across a variety of datasets and models while maintaining our target latency (under P90 200 milliseconds). These benchmarks aren’t designed for evaluating ML models. A model’s impact on search quality varies with multiple factors, including the dataset. Disk mode’s optimizations under the hood When you configure a k-NN index to run on disk mode , OpenSearch automatically applies a quantization technique, compressing vectors as they’re loaded to build a compressed index. By default, disk mode converts each full-precision vector—a sequence of hundreds to thousands of dimensions, each stored as 32-bit numbers—into binary vectors, which represent each dimension as a single-bit. This conversion results in a 32 times compression rate, enabling the engine to build an index that is 97% smaller than one composed of full-precision vectors. A right-sized cluster will keep this compressed index in memory. Compression lowers cost by reducing the memory required by the vector engine, but it sacrifices accuracy in return. Disk mode recovers accuracy, and therefore search quality, using a two-step search process. The first phase of the query execution begins by efficiently traversing the compressed index in memory for candidate matches. The second phase uses these candidates to oversample corresponding full-precision vectors. These full-precision vectors are stored on disk in a format designed to reduce I/O and optimize disk retrieval speed and efficiency. The sample of full-precision vectors is then used to augment and re-score matches from phase one (using exact k-NN ), thereby recovering the search quality loss attributed to compression. Disk mode’s higher latency relative to memory mode is attributed to this re-scoring process, which requires disk access and additional computation. Early customer successes Customers are already running the vector engine in disk mode. In this section, we share testimonials from early adopters. Asana is improving search quality for customers on their work management platform by phasing in semantic search capabilities through OpenSearch’s vector engine. They initially optimized the deployment by using product quantization to compress indexes by 16 times. By switching over to the disk-optimized configurations, they were able to potentially reduce cost by another 33% while maintaining their search quality and latency targets. These economics make it viable for Asana to scale to billions of vectors and democratize semantic search throughout their platform. DevRev bridges the fundamental gap in software companies by directly connecting customer-facing teams with developers. As an AI-centered platform, it creates direct pathways from customer feedback to product development, helping over 1,000 companies accelerate growth with accurate search, fast analytics, and customizable workflows. Built on large language models (LLMs) and Retrieval Augmented Generation (RAG) flows running on OpenSearch’s vector engine, DevRev enables intelligent conversational experiences. “With OpenSearch’s disk-optimized vector engine, we achieved our search quality and latency targets with 16x compression. OpenSearch offers scalable economics for our multi-billion vector search journey.” – Anshu Avinash, Head of AI and Search at DevRev. Get started with disk mode on the OpenSearch Vector Engine First, you need to determine the resources required to host your index. Start by estimating the memory required to support your disk-optimized k-NN index (with the default 32 times compression rate) using the following formula: Required memory (bytes) = 1.1 x ((vector dimension count)/8 + 8 x m) x (vector count) For instance, if you use the defaults for Amazon Titan Text V2 , your vector dimension count is 1024. Disk mode uses the HNSW algorithm to build indexes, so “m” is one of the algorithm parameters, and it defaults to 16. If you build an index for a 1-billion vector corpus encoded by Amazon Titan Text, your memory requirements are 282 GB. If you have a throughput-heavy workload, you need to make sure your domain has sufficient IOPs and CPUs as well. If you follow deployment best practices, you can use instance store and storage performance optimized instance types, which will generally provide you with sufficient IOPs. You should always perform load testing for high-throughput workloads, and adjust the original estimates to accommodate for higher IOPs and CPU requirements. Now you can deploy an OpenSearch 2.17+ domain that has been right-sized to your needs. Create your k-NN index with the mode parameter set to on_disk , and then ingest your data . If you already have a k-NN index running on the default in_memory mode, you can convert it by switching the mode to on_disk followed by a reindex task. After the index is rebuilt, you can downsize your domain accordingly. Conclusion In this post, we discussed how you can benefit from running the OpenSearch Vector Engine on disk mode, shared customer success stories, and provided you tips on getting started. You’re now set to run the OpenSearch Vector Engine at as low as a third of the cost. To learn more, refer to the documentation . About the Authors Dylan Tong is a Senior Product Manager at Amazon Web Services. He leads the product initiatives for AI and machine learning (ML) on OpenSearch including OpenSearch’s vector database capabilities. Dylan has decades of experience working directly with customers and creating products and solutions in the database, analytics and AI/ML domain. Dylan holds a BSc and MEng degree in Computer Science from Cornell University. Vamshi Vijay Nakkirtha is a software engineering manager working on the OpenSearch Project and Amazon OpenSearch Service. His primary interests include distributed systems.
DevRev Frequently Asked Questions (FAQ)
When was DevRev founded?
DevRev was founded in 2020.
Where is DevRev's headquarters?
DevRev's headquarters is located at 300 Hamilton Avenue, Palo Alto.
What is DevRev's latest funding round?
DevRev's latest funding round is Series A.
How much did DevRev raise?
DevRev raised a total of $150.8M.
Who are the investors of DevRev?
Investors of DevRev include Khosla Ventures, Mayfield, U First Capital, Param Hansa Values and Alumni Ventures.
Who are DevRev's competitors?
Competitors of DevRev include Creatio and 4 more.
Loading...
Compare DevRev to Competitors
Banza specializes in digital transformation and business process automation within the banking and retail sectors. The company offers customer relationship management (CRM) and business process management (BPM) solutions to streamline sales, marketing, and customer service processes. Banza primarily serves the banking and retail industries with comprehensive solutions for credit management, sales, customer service, and omnichannel customer interactions. It was founded in 2020 and is based in Uzhhorod, Ukraine.
Unify focuses on providing solutions for go-to-market teams. It operates within the technology and sales sector. The company offers a range of services, including understanding buyer intent, finding contacts, and sending AI-personalized emails. It caters to growth, marketing, and sales teams across various industries. It was founded in 2023 and is based in San Francisco, California.
Five Elements Labs focuses on Web3 marketing technology within the blockchain sector. Its main offering, Tide, is a marketing suite designed to engage and reward online communities, enhancing customer engagement and retention. The company primarily serves businesses looking to improve their marketing strategies in the Web3 space. It was founded in 2022 and is based in Milan, Italy.
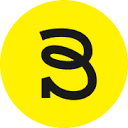
Bizzabo provides event management software for B2B conferences and events. The company offers a platform that includes solutions for registration, audience engagement, networking, marketing, and data analytics. Bizzabo's services cater to various sectors, including corporations, agencies, nonprofits, higher education institutions, and associations. It was founded in 2011 and is based in New York, New York.
Conference Compass develops event technology, creating mobile and web applications for in-person, virtual, and hybrid events. The company provides an event engagement platform that offers content and captures analytics. Conference Compass serves associations, professional conference organizers, corporate meeting planners, and event tech suppliers. It was founded in 2010 and is based in The Hague, Netherlands.
Integry is a technology company that focuses on providing integration solutions. The company offers a platform that allows users to embed integrations into their applications, enabling data push and pull from hundreds of apps without the need for coding. The primary sectors that Integry serves include software businesses and enterprises that require seamless data integration. It was founded in 2017 and is based in Walnut, California.
Loading...