
DataStax
Founded Year
2010Stage
Secondary Market | AliveTotal Raised
$342.27MMosaic Score The Mosaic Score is an algorithm that measures the overall financial health and market potential of private companies.
-26 points in the past 30 days
About DataStax
DataStax specializes in providing a comprehensive generative AI stack for the development and deployment of production-ready applications within the technology sector. The company offers a RAG API that supports both vector and structured data, ensuring secure, compliant, and scalable solutions that are integrated with leading AI ecosystem partners. DataStax primarily caters to developers and enterprises looking to leverage generative AI technologies across various cloud platforms. It was founded in 2010 and is based in Santa Clara, California.
Loading...
ESPs containing DataStax
The ESP matrix leverages data and analyst insight to identify and rank leading companies in a given technology landscape.
The NoSQL database market revolves around the development, provision, and adoption of non-relational database management systems. NoSQL databases are designed to handle large volumes of unstructured or semi-structured data, offering scalability, high performance, and flexibility compared to traditional relational databases. The market encompasses a variety of NoSQL database technologies, including…
DataStax named as Leader among 15 other companies, including Microsoft Azure, Oracle, and Cloudera.
Loading...
Expert Collections containing DataStax
Expert Collections are analyst-curated lists that highlight the companies you need to know in the most important technology spaces.
DataStax is included in 2 Expert Collections, including Unicorns- Billion Dollar Startups.
Unicorns- Billion Dollar Startups
1,257 items
Tech IPO Pipeline
543 items
DataStax Patents
DataStax has filed 21 patents.
The 3 most popular patent topics include:
- database management systems
- data management
- binary trees
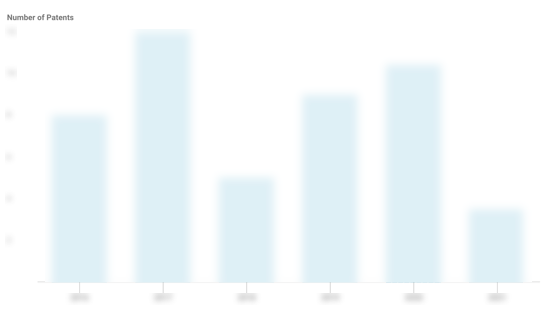
Application Date | Grant Date | Title | Related Topics | Status |
---|---|---|---|---|
10/14/2022 | 8/13/2024 | Cloud computing, Configuration management, Rotating disc computer storage media, Cloud platforms, Cloud storage | Grant |
Application Date | 10/14/2022 |
---|---|
Grant Date | 8/13/2024 |
Title | |
Related Topics | Cloud computing, Configuration management, Rotating disc computer storage media, Cloud platforms, Cloud storage |
Status | Grant |
Latest DataStax News
Dec 19, 2024
Published on December 19, 2024 Share by Sagar Sharma DataStax is reshaping the AI landscape by redefining how enterprises build and deploy intelligent agents. We are witnessing a shift where building AI agents is no longer a complex task, and almost everyone can develop their own agents without requiring much technical knowledge. At the RAG++ Bangalore event, Mukundha Madhavan , technology lead at DataStax, told AIM that AI agents are no longer just theoretical constructs or experimental projects. “They are becoming integral to how businesses operate, and the need for robust, scalable solutions has never been greater.” Madhavan pointed out a growing trend in which companies are seen moving from just experimenting with AI in proof-of-concept projects to full-scale production environments. Simplifying Agent Development with Langflow At the heart of DataStax’s innovation is Langflow – a low-code app builder for RAG and multi-agent AI applications. “Langflow lets developers visually create workflows simply by dragging and dropping components; it uses AI development as seamlessly as possible,” Madhavan further explained. This kind of approach relieves developers from the burden of learning extensive coding skills because they can invest their time working on the function rather than dealing with technical barriers. Langflow has included various kinds of agents, including task-oriented ones that are good at performing specific functions. DataStax released multi-agent orchestration in Langflow 1.1 to enable multiple agents to work together on complex tasks. This actually weighs quite a lot in terms of the current demand for AI systems capable of dealing with dynamic and multifaceted workflows. Moreover, the conditional routing and multimodal inputs in Langflow allow developers to design workflows that dynamically change in response to either the nature of the inputs or certain conditions. Langflow itself has been used to create AI shopping assistants that interconnect customer information with product catalogues in real-time. However, NVIDIA collaboration has played a huge part in improving the platform’s performance levels. Utilising NVIDIA’s optimised hardware and services, Langflow accelerates data processing by 19 times over traditional provisions. “There is a lot of dynamism in the collaboration with NVIDIA. It is not only speed but more importantly, it also enables more advanced AI capabilities, which would be impossible otherwise,” Madhavan said. Where JVector Comes into the Picture? JVector is designed for speed and flexibility. Using modern graph algorithms inspired by DiskANN (Disk Aware Approximate Nearest Neighbor) algorithms allows JVector to handle large datasets swiftly while maintaining high recall and low latency. In a study done with Deep100M datasets , JVector significantly outperformed classical engines like Lucene. This makes it particularly suitable for large data application scenarios or cases that require real-time search capability. One of the main features of JVector is its disk-aware design. It can process large datasets that exceed memory capacity. Via DiskANN-style search and product quantisation, JVector compresses vectors into memory-efficient formats while minimising input or output operations during query execution. This feature is particularly relevant for retrieval-augmented generation (RAG) workflows in which large-scale embeddings are leveraged to give contextually relevant information to generative AI models. JVector allows simultaneous index building and scales with up to 32 threads. This enables developers to update indexes on the fly without affecting active queries, which is critical for real-time AI applications that constantly require data updates. Moreover, utilising the Panama SIMD API, it speeds up indexing and search operations by by processing multiple data points simultaneously. This leads to faster query responses and improved throughput for enterprise-level applications. “JVector is not just a tool – it’s a foundation for creating intelligent systems that can understand and respond to complex queries in real-time,” Madhavan aptly said. The Role of Real-Time Data A key differentiator for DataStax is its foundation in real-time data management. Built on Apache Cassandra, the platform addresses one of the most pressing challenges in AI development: data fragmentation. “The most common issue I come across when talking to developers and customers is fragmented data sources,” Madhavan noted. “Without a unified view of data, building effective AI agents becomes nearly impossible.” According to Kohezion, almost 80% of organisations have over half of their data spread across various clouds or infrastructures. Companies can enhance their workflows and boost productivity by bringing these datasets together into one system with real-time access. DataStax’s real-time data architecture ensures that agents have immediate access to relevant information. This capability is crucial for applications ranging from customer service chatbots to predictive maintenance systems in industrial settings. This capability has already transformed industries. For example, PhysicsWallah uses DataStax’s platform to deliver personalised learning experiences to over 20 million students in India while managing a 50x surge in traffic without downtime. DataStax is focused on expanding the potential of AI agents. The company is investigating sophisticated multi-agent systems in which agents not only work together but also learn from one another in real time. “The future lies in ecosystems of intelligent agents that can adapt dynamically to changing environments,” Madhavan said while sharing his vision. 📣 Want to advertise in AIM? Book here
DataStax Frequently Asked Questions (FAQ)
When was DataStax founded?
DataStax was founded in 2010.
Where is DataStax's headquarters?
DataStax's headquarters is located at 2755 Augustine Drive, Santa Clara.
What is DataStax's latest funding round?
DataStax's latest funding round is Secondary Market.
How much did DataStax raise?
DataStax raised a total of $342.27M.
Who are the investors of DataStax?
Investors of DataStax include CrossWork, Crosslink Capital, Meritech Capital Partners, Goldman Sachs Asset Management, EDBI and 22 more.
Who are DataStax's competitors?
Competitors of DataStax include Aerospike, CrateDB, VectorShift, VAST Data, ScyllaDB and 7 more.
Loading...
Compare DataStax to Competitors

Cockroach Labs focuses on the development of distributed structured query language (SQL) databases. It provides CockroachDB, offering features such as elastic scaling, cloud-native compatibility, built-in survivability, and consistent SQL, primarily serving industries such as finance, gaming, manufacturing and logistics, media and streaming, retail, and e-commerce. It was founded in 2015 and is based in New York, New York.
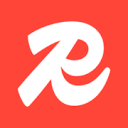
Redis is a real-time data platform that focuses on providing in-memory data structure storage and enterprise scaling solutions. The company offers products such as an in-memory database for caching and streaming, a fully managed cloud service integrated with major cloud providers, and self-managed software designed for enterprise needs. Redis primarily serves sectors that require high-performance data operations, such as financial services, gaming, healthcare, and retail. It was founded in 2011 and is based in San Francisco, California.

Aerospike specializes in data management solutions within the NoSQL database sector. The company offers a distributed NoSQL database designed for high-speed reads and writes, capable of scaling from gigabytes to petabytes without performance trade-offs. Aerospike's database supports applications requiring consistent, sub-millisecond latency at a massive scale. Aerospike was formerly known as Citrusleaf. It was founded in 2009 and is based in Mountain View, California.
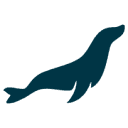
MariaDB is a cloud database company that specializes in open-source database products and services for various business sectors. The company offers enterprise and community versions of its database server, which support transactional, analytical, and mixed workloads for relational and JSON data models. Its services include technical support, database migration, remote database administration, consulting, and training. MariaDB was formerly known as SkySQI. It was founded in 2010 and is based in Milpitas, California.
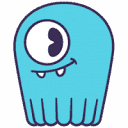
ScyllaDB provides NoSQL database solutions for various industries. The company offers a distributed database that handles data across different infrastructures. ScyllaDB's products are used in sectors such as technology, finance, and entertainment, with options for cloud-native and on-premises databases. It was founded in 2013 and is based in Sunnyvale, California.
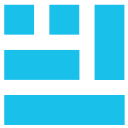
CrateDB operates as an enterprise database optimized for time series, documents, and vector data workloads. The company offers a distributed structured query language (SQL) database that enables complex queries on various data types and integrates with artificial intelligence (AI) and machine learning (ML) frameworks. It primarily serves sectors that require real-time analytics and data management, such as AI/ML industries. The company was founded in 2013 and is based in Redwood City, California.
Loading...